The BEA 2024 Shared Task on the Multilingual Lexical Simplification Pipeline
Jun 20, 2024·
,,,,,,,,,,,,,,,,,,,,·
0 min read
Matthew Shardlow

Fernando Alva-Manchego
Riza Batista-Navarro
Stefan Bott
Saul Calderon Ramirez
Rémi Cardon
Thomas François
Akio Hayakawa
Andrea Horbach
Anna Hülsing
Yusuke Ide
Joseph Marvin Imperial
Adam Nohejl
Kai North
Laura Occhipinti
Nelson Peréz Rojas
Nishat Raihan
Tharindu Ranasinghe
Martin Solis Salazar
Sanja Štajner
Marcos Zampieri
Horacio Saggion
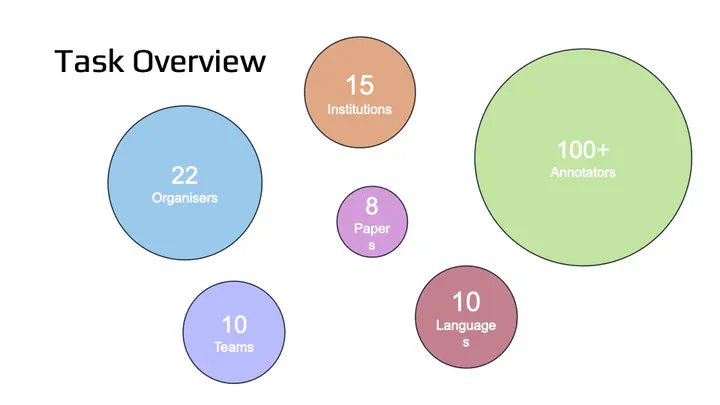
Abstract
We report the findings of the 2024 Multilingual Lexical Simplification Pipeline shared task. We released a new dataset comprising 5,927 instances of lexical complexity prediction and lexical simplification on common contexts across 10 languages, split into trial (300) and test (5,627). 10 teams participated across 2 tracks and 10 languages with 233 runs evaluated across all systems. Five teams participated in all languages for the lexical complexity prediction task and 4 teams participated in all languages for the lexical simplification task. Teams employed a range of strategies, making use of open and closed source large language models for lexical simplification, as well as feature-based approaches for lexical complexity prediction. The highest scoring team on the combined multilingual data was able to obtain a Pearson’s correlation of 0.6241 and an ACC@1@Top1 of 0.3772, both demonstrating that there is still room for improvement on two difficult sub-tasks of the lexical simplification pipeline.
Type
Publication
BEA 2024